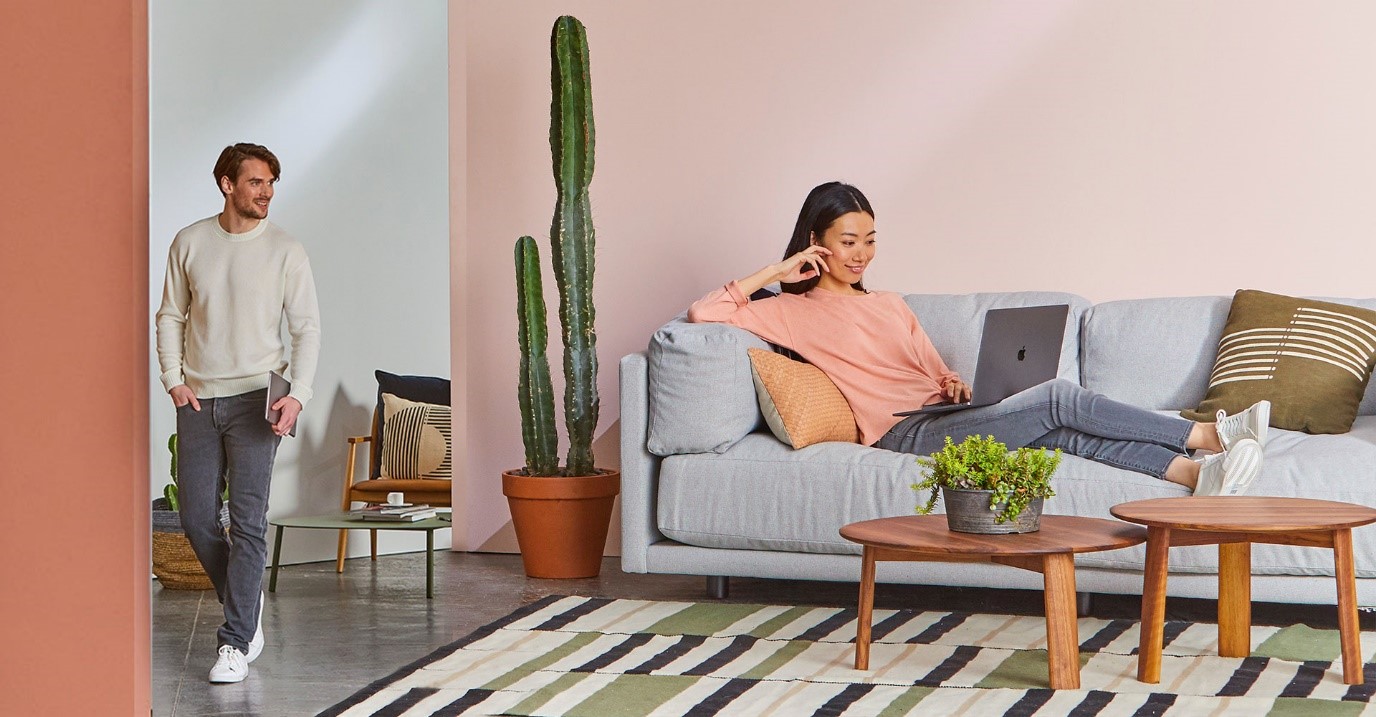
AI-driven Sentiment Analysis: Uncovering hidden insights from survey responses
Introduction:
In the age of big data, organizations are inundated with vast amounts of customer feedback and survey responses. Extracting meaningful insights from this data can be a challenging task. However, with advancements in Artificial Intelligence (AI), specifically in sentiment analysis, organizations can now uncover valuable insights hidden within survey responses. In this blog post, we will explore the power of AI-driven sentiment analysis and how it enables organizations to gain a deeper understanding of customer sentiments and preferences. Let's dive in!
What is Sentiment Analysis?
Sentiment analysis, also known as opinion mining, is a technique that uses Natural Language Processing (NLP) and machine learning algorithms to analyze and determine the sentiment expressed in text data. It helps classify text as positive, negative, or neutral, providing valuable insights into customer emotions, attitudes, and opinions.
The Role of AI in Sentiment Analysis:
AI plays a crucial role in sentiment analysis by leveraging advanced algorithms and machine learning models to automatically analyze text and extract sentiment. By training on vast amounts of labeled data, AI models can learn to recognize patterns, understand context, and accurately classify sentiments across different domains and languages.
Benefits of AI-Driven Sentiment Analysis in Surveys:
- Uncovering Hidden Insights: Traditional manual analysis of survey responses can be time-consuming and prone to human biases. AI-driven sentiment analysis enables organizations to uncover hidden insights by automatically processing large volumes of text data and extracting sentiment at scale.
- Real-Time Analysis: AI-powered sentiment analysis provides real-time insights, allowing organizations to promptly address customer concerns, identify emerging trends, and make data-driven decisions in a timely manner.
- Improved Customer Understanding: By analyzing sentiments expressed in survey responses, organizations gain a deeper understanding of customer preferences, pain points, and satisfaction levels. This understanding can inform product development, marketing strategies, and customer experience improvements.
- Enhanced Decision Making: AI-driven sentiment analysis provides valuable inputs for decision-making processes across various departments, including marketing, product management, customer support, and sales. It enables organizations to align their strategies and actions with customer sentiments, ultimately driving customer satisfaction and loyalty.
Implementing AI-Driven Sentiment Analysis:
- Data Collection and Preparation: Gather survey responses and ensure the data is clean and properly formatted. Preprocessing steps, such as removing stop words, stemming, and normalization, may be required to enhance analysis accuracy.
- Training and Fine-Tuning: Train an AI model using labeled data, where sentiments are manually annotated. Fine-tune the model to align with specific domain or industry jargon, ensuring accurate sentiment classification.
- Sentiment Analysis Algorithms: Select and apply appropriate sentiment analysis algorithms, such as Naive Bayes, Support Vector Machines (SVM), or Recurrent Neural Networks (RNN), depending on the complexity of the analysis task.
- Evaluation and Validation: Evaluate the performance of the sentiment analysis model using metrics like accuracy, precision, recall, and F1 score. Validate the model's results by comparing them with human-annotated sentiments for a subset of data.
Challenges and Considerations:
- Language and Context: Sentiment analysis faces challenges with languages, dialects, slang, and cultural nuances. Consider these factors when training and applying sentiment analysis models to ensure accurate results.
- Domain-Specific Analysis: Sentiment analysis models trained on general data may not perform well in domain-specific contexts. Fine-tuning the models with industry-specific data can help improve accuracy and relevance.
- Feedback Loop and Model Iteration: Continuously collect feedback on sentiment analysis results and iteratively refine the models to enhance their performance over time.
Conclusion:
AI-driven sentiment analysis revolutionizes the way organizations extract insights from survey responses. By leveraging the power of AI and NLP, organizations can uncover hidden sentiments, gain a comprehensive understanding of customer preferences, and make data-driven decisions. Implementing AI-driven sentiment analysis involves careful data collection, model training, algorithm selection, and validation. By overcoming challenges and considering domain-specific factors, organizations can harness the full potential of sentiment analysis and unlock valuable insights from survey responses